The Impact of Machine Learning Journal Articles on Business Innovation
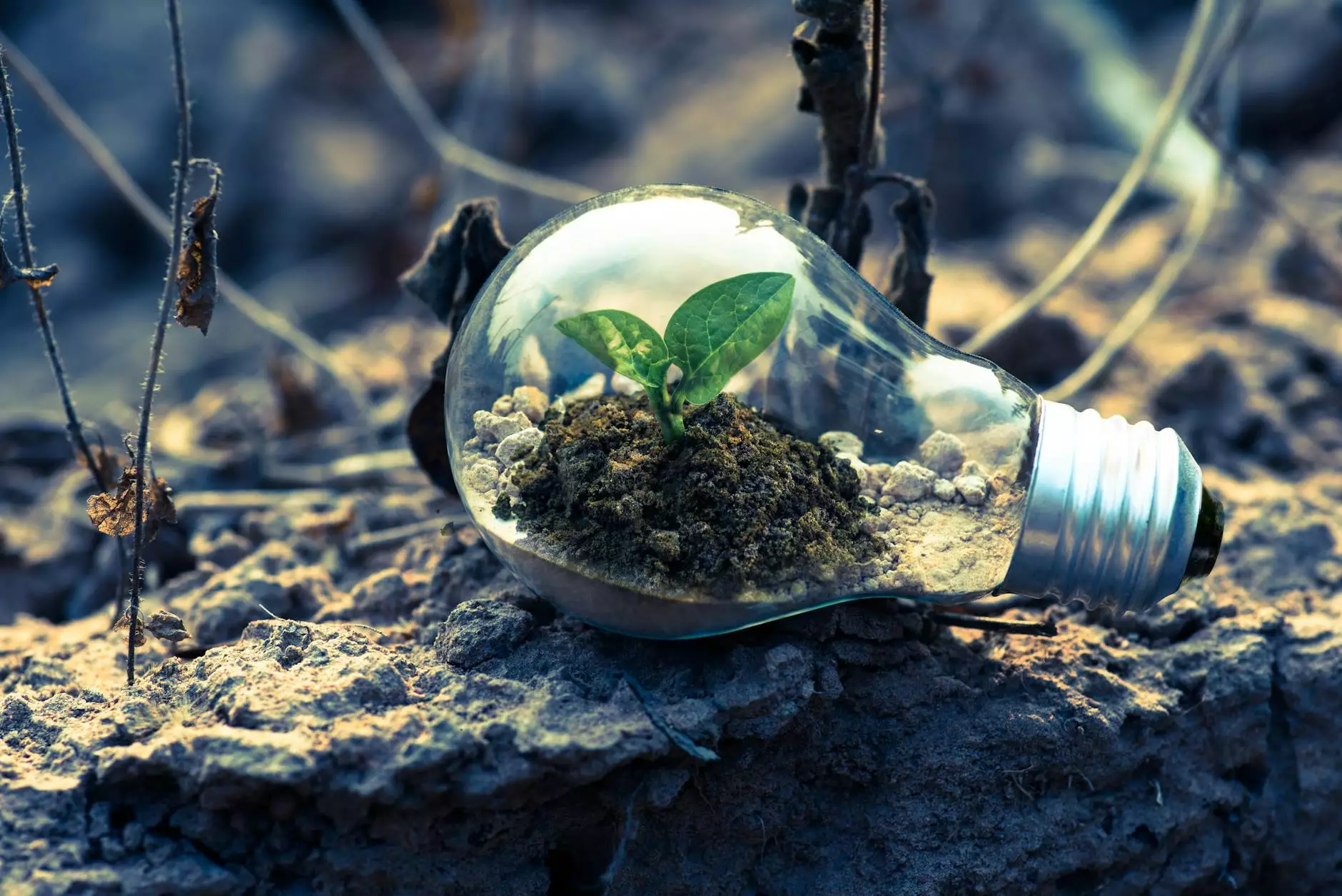
In the contemporary business landscape, the profound influence of technology cannot be overstated. Among these technological advancements, machine learning stands out as a pivotal force driving innovation and operational efficiency. This article delves into the realm of machine learning journal articles, exploring their significance in propelling business strategies, enhancing decision-making, and fostering the development of cutting-edge solutions.
Understanding Machine Learning
Machine learning, a subset of artificial intelligence (AI), involves the development of algorithms that enable computers to learn from and make predictions based on data. Unlike traditional programming, where explicit instructions are given, machine learning allows systems to adaptively improve their performance as they are exposed to more data.
The essence of machine learning lies in its ability to analyze vast amounts of information, identifying patterns and uncovering insights that would be impossible or time-consuming for humans to detect. This capability poses a transformative opportunity for businesses across various sectors, from healthcare to finance, as they seek to leverage data for competitive advantage.
The Role of Machine Learning Journal Articles in Business
Machine learning journal articles serve as a crucial resource for professionals and researchers alike. These documents provide valuable insights into the latest research findings, methods, and applications of machine learning, contributing to the continuous evolution of the field. Here are several key ways in which these articles impact businesses:
1. Informing Business Strategies
Access to the latest research through machine learning journal articles enables businesses to stay informed about cutting-edge developments. By understanding emerging trends, companies can adapt their strategies to incorporate machine learning tools that enhance operational efficiency and customer satisfaction.
2. Facilitating Data-Driven Decision Making
Data-driven decision-making is at the heart of modern business management. Machine learning journal articles elucidate methodologies that organizations can apply to analyze data effectively. By utilizing these findings, businesses can make informed choices about product development, marketing initiatives, and resource allocation, ultimately leading to improved ROI.
3. Encouraging Collaboration and Innovation
The body of knowledge disseminated through machine learning journal articles encourages collaboration among researchers, practitioners, and industry leaders. This synergy catalyzes innovative solutions that address complex business challenges. As companies engage with academic research, they can identify partnership opportunities that drive growth.
Key Areas of Focus in Machine Learning Research
Various areas of machine learning research are particularly relevant to business applications:
- Predictive Analytics: Leveraging historic data to forecast future trends and behaviors.
- Natural Language Processing (NLP): Enabling machines to understand and interpret human language, enhancing customer interactions.
- Computer Vision: Allowing systems to interpret and process visual information, applicable in sectors like retail and security.
- Reinforcement Learning: A method of teaching algorithms through trial and error, useful for optimizing complex systems.
- Unsupervised Learning: Identifying patterns in data without labeled outputs, important for market segmentation and anomaly detection.
The Importance of High-Quality Research Articles
While numerous resources exist regarding machine learning, the quality of the research presented in journal articles is paramount. High-quality articles undergo rigorous peer review, ensuring that the information is accurate, reliable, and relevant to current business needs. As such, businesses must prioritize sourcing knowledge from credible academic sources.
Moreover, the credibility of these machine learning journal articles contributes to their acceptance in the academic and business communities. Incorporating well-established findings can significantly bolster organizational initiatives and foster trust among stakeholders.
Applications of Machine Learning in Business
The applications of machine learning in business are extensive and varied, reflecting its versatility. Below are some key applications that exemplify how machine learning can transform traditional business operations:
1. Product Recommendations
Online retail platforms utilize machine learning algorithms to analyze customer preferences and behaviors, providing personalized product recommendations. This approach enhances the customer experience and significantly increases conversion rates.
2. Fraud Detection
In the finance sector, machine learning models are deployed to detect fraudulent transactions by identifying unusual patterns indicative of fraud. Continuous learning from new transaction data allows these systems to evolve alongside emerging fraud tactics.
3. Supply Chain Optimization
Businesses use machine learning for demand forecasting, inventory management, and route optimization. By analyzing data across various touchpoints, organizations can streamline supply chain operations, reduce costs, and enhance service delivery.
4. Customer Segmentation
By leveraging unsupervised learning techniques, companies can segment their customer base into distinct groups based on purchasing behavior, preferences, and demographics. This segmentation facilitates targeted marketing strategies and personalized offerings, improving customer retention.
5. Talent Acquisition
Machine learning algorithms are revolutionizing the hiring process by analyzing resumes and predicting candidate suitability based on various parameters. This not only speeds up recruitment but also helps in aligning the right talent with organizational goals.
Emerging Trends in Machine Learning
The field of machine learning is ever-evolving, with new trends continually reshaping the business landscape. Some notable trends include:
- Explainable AI: As machine learning models become more complex, the demand for transparency in AI decisions grows. Businesses are increasingly focusing on developing explainable models that can offer insights into their decision-making processes.
- AutoML: Automated machine learning simplifies the process of building and deploying machine learning models, making it accessible to non-experts and compressing development timelines.
- Federated Learning: This approach allows multiple parties to collaborate in training machine learning models without sharing sensitive data, ensuring data privacy while enhancing model accuracy.
- Real-Time Analytics: With the growth of IoT devices and streaming data, businesses are investing in machine learning solutions that enable real-time data analysis and immediate decision-making.
- Integration with Edge Computing: Combining machine learning with edge computing reduces latency and enhances real-time processing capabilities, crucial for applications like autonomous vehicles and smart cities.
Conclusion
The integration of machine learning into business practices is no longer optional; it has become a necessity for organizations aiming to thrive in an increasingly competitive environment. By tapping into the wealth of knowledge offered by machine learning journal articles, businesses can unlock transformative insights, driving innovation and efficiency.
From enhancing customer experiences to streamlining operations, the potential applications of machine learning are vast and impactful. As businesses continue to harness the power of this technology, the future will undoubtedly be shaped by those who proactively engage with and contribute to the ongoing research in the domain of machine learning.